When
Where
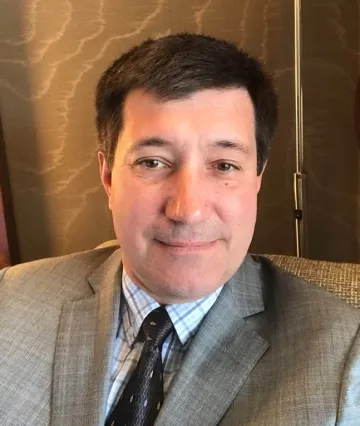
Presenter: Dr. Sergei Tretiak,
Theoretical Division, Center for Integrated Nanotechnologies,
Los Alamos National Laboratory
Abstract:
Machine learning (ML) became a premier tool for modeling chemical processes and materials properties. Generally, ML provides a surrogate model trained on the dataset of some reference data, establishing a relationship between structure and underlying chemical properties. For instance, ML interatomic potentials have become an efficient alternative to computationally expensive quantum chemistry simulations.
In practice, however, developing models for reactive chemistry requires prior knowledge of reaction networks to generate fitting data and refitting to extensive datasets for each new application. To address this challenge, we develop a general reactive ML interatomic potential through unbiased active learning with an atomic configuration sampler inspired by nanoreactor molecular dynamics. The resulting model is then applied to study five distinct condensed-phase reactive chemistry systems: carbon solid-phase nucleation, graphene ring formation from acetylene, biofuel additives, combustion of methane and the spontaneous formation of glycine from early-earth small molecules.
In all cases, the results closely match experiment and/or previous studies using traditional model chemistry methods. Importantly, the model does not need to be refit for each application, enabling high throughput in silico reactive chemistry experimentation. In another example, we take the structure of semiempirical quantum mechanics methods to construct dynamically responsive Hamiltonians. By replacing the static Hamiltonian’s parameters with ML dynamic values inferred from the local environment, we greatly improve the accuracy of the reduced quantum mechanical framework. Developed models are simultaneously accurate, transferable, and interpretable, being particularly useful for non-equilibrium chemical dynamics simulations. Altogether, explosive growth of user-friendly ML frameworks, designed for chemistry, demonstrates that the field is evolving towards physics-based models augmented by data science.