When
Abstract
New efficient and cost-effective materials are needed for numerous sustainability and energy applications, such as thermoelectric materials. However, advancements in this field are often hindered by the scarcity of available data and the significant effort required to acquire new data, both computationally and experimentally. For such applications, reliable surrogate models that help guide materials space exploration using easily accessible materials properties are urgently needed. Here, I present a general, high-throughput framework for calculating the thermal transport properties of a material from first-principles1. By using the workflow to calculate the structural, harmonic, and anharmonic properties of a material I am able to create a set of physically meaningful descriptors for its thermal conductivity, κL. Using these descriptors, I use symbolic regression2 to build an analytical model that describes κL, and then extract out the most important input properties using a variance-based sensitivity analysis3 . I then use this example to highlight the potential power GPUs for these types of applications and the challenges of porting codes onto them. Utilizing the information gained from the the AI models, I screen over a set of 732 materials and find 80 ultra-insulating materials in the region of materials space that is likely to contain new thermal insulators4. Finally, I confirm four of these predictions by calculating their thermal conductivity using the ab initio Green-Kubo technique1,5.
[1] Knoop, F.; Purcell, T. A. R.; Scheffler, M.; and Carbogno, C. J. Open Source Softw. 2020, 5, 2671
[2] Purcell, T. A. R.; Scheffler, M.; Carbogno, C.; and Ghiringhelli, L. M. J. Open Source Softw. 2022, 7, 3960
[3] Kucherenko, S.; Tarantola, S.; and Annoni, P. Comput. Phys. Commun. 2012, 183, 937
[4] Purcell, T. A. R.; Scheffler, M.; Ghiringhelli, L. M.; and Carbogno, C. npj Comput. Mater.2023 9 112
[5] Knoop, F.; Purcell, T. A. R.; Scheffler, M.; and Carbogno, C. Phys. Rev. Lett. 2023, 130 236301
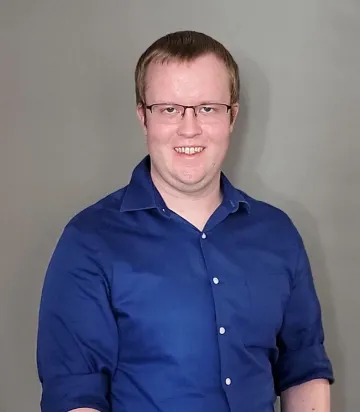
Presenter
Dr. Thomas Purcell
Assistant Professor, Chemistry and Biochemistry, The University of Arizona
Biosketch
Thomas Purcell is one of the newest faculty members of CBC at the University of Arizona, focusing on applying artificial intelligence and data science for functional materials discovery. He began his scientific career at New York University where he received his B.S. in Chemistry, before getting his Ph.D. at Northwestern University under Prof. Tamar Seideman. During his Ph.D. he focused on understanding the optical coupling between quantum emitters and plasmonic nanoparticles using classical and semicalssical electrodynamics methods. After his Ph.D. he joined the Theory Department (now the NOMAD Laboratory) of the Fritz-Haber-Insitut der Max-Plack-Gesellschaft (FHI) as an Alexander von Humboldt Postdoctoral Fellow, and later became a Group Leader in the same department. At the FHI, Thomas began his work on high-throughput computational workflows and AI for materials discovery that he is continuing at the University of Arizona.